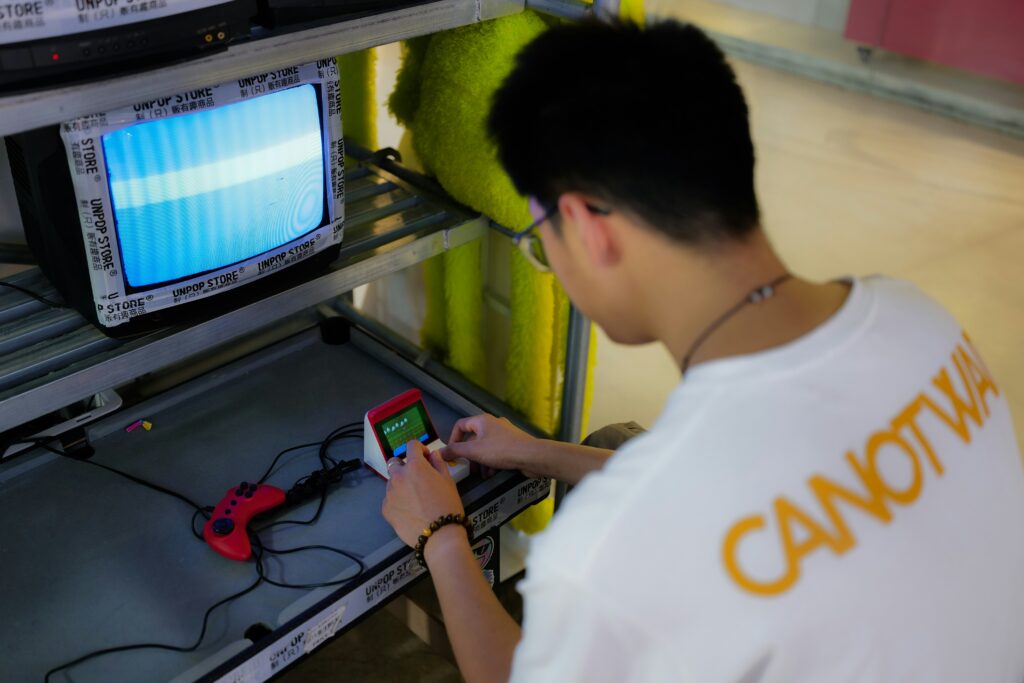
Introduction
In the era of Industry 5.0, human machine collaboration is more critical than ever. Traditional manufacturing systems focused heavily on automation, but the latest advancements emphasize human-centric manufacturing, integrating workers, machines, and logistics in real-time production environments. However, human performance varies due to learning and forgetting effects, which pose challenges for scheduling and task coordination.
This blog explores an adaptive human machine logistics scheduling framework that accounts for worker efficiency fluctuations, enhancing multi-resource collaboration. By leveraging real-time task-driven scheduling, manufacturing—supported by Siemens and Schneider Electric—can achieve greater efficiency while reducing completion time, energy consumption, and delays
The Rise of Human Machine Manufacturing
Industry 5.0 shifts the focus from automation alone to human machine synergy, where workers actively engage with intelligent systems to boost efficiency and sustainability. Unlike traditional scheduling methods that primarily consider machine and logistics resources, this approach integrates human factors, acknowledging the dynamic nature of worker efficiency.
Workers develop skills over time, increasing productivity through learning effects. However, interruptions lead to forgetting effects, negatively impacting efficiency. Accurately modeling these variations is crucial for optimizing scheduling decisions in smart workshops.
Challenges in Human Machine Logistics Collaboration
In real-time smart workshops, scheduling must accommodate constant fluctuations in resource availability, worker proficiency, and logistical constraints. The key challenges include:
- Dynamic worker efficiency: Learning improves performance, while forgetting reduces effectiveness.
- Task allocation complexity: Machines, workers, and logistics units must coordinate in real-time.
- Energy consumption concerns: Smart manufacturing aims to optimize performance while minimizing resource usage.
Most current scheduling models ignore worker variability, relying solely on machines and logistics. This oversight results in suboptimal task allocation, affecting production efficiency and sustainability.
Human Machine Proposed Solution: Real-Time Collaborative Scheduling
This study introduces a real-time task-driven human machine logistics scheduling framework that enhances multi-resource coordination while accounting for learning and forgetting effects.
1. Human Machine Learning-Forgetting Model for Dynamic Worker Efficiency
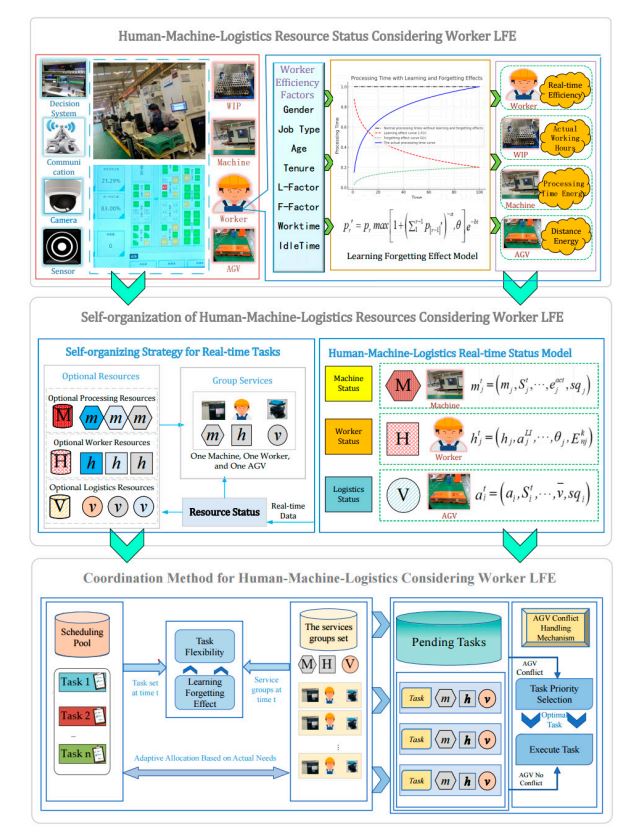
A mathematical model quantifies worker performance, adjusting scheduling decisions based on real-time efficiency variations. The model dynamically updates:
- Worker state: Learning and forgetting rates determine task proficiency.
- Machine state: Task duration adapts to worker efficiency.
- Logistics synchronization: Material handling aligns with optimized workflow.
2. Self-Organizing Multi-Resource Groups
Unlike static scheduling systems, this framework allows human machine logistics resources to form adaptive groups based on task requirements. Through self-organization, resources dynamically regroup to maximize task efficiency.
3. Task Slack-Based Matching for Optimal Scheduling
The system utilizes task slack analysis to allocate resources dynamically. Urgent tasks receive priority scheduling, while tasks with flexibility are assigned to energy-efficient configurations.
Case Study: Applying the Framework in Smart Manufacturing
To validate this method, an engineering case study was conducted in a hydraulic valve block production workshop. Using Siemens Tecnomatix Plant Simulation software, various scheduling scenarios were tested. The results demonstrated significant improvements:
- Completion time reduction: Decreased by 10% on average.
- Energy consumption optimization: Lowered by 8%.
- Delay minimization: Reduced by 70%.
These findings confirm the effectiveness and adaptability of the proposed framework, proving its value in dynamic production environments.
Conclusion
The integration of learning and forgetting effects into real-time human machine logistics scheduling marks a revolutionary step toward Industry 5.0 adoption. By dynamically adjusting scheduling strategies based on worker efficiency, smart workshops achieve:
- Faster task completion
- Optimized energy usage
- Improved production accuracy
As manufacturing continues evolving, adaptive scheduling models will play a pivotal role in ensuring sustainable, intelligent, and human-centric production systems.
Click here to learn more about nanorobots in medicine.
Reference
Yang, W.; Li, S.; Luo, G.; Li, H.; Wen, X. A Real-Time Human Machine Logistics Collaborative Scheduling Method Considering Workers’ Learning and Forgetting Effects. Applied System Innovation 2025, 8, 40. https://doi.org/10.3390/asi8020040.
License: Creative Commons Attribution (CC BY) https://creativecommons.org/licenses/by/4.0/
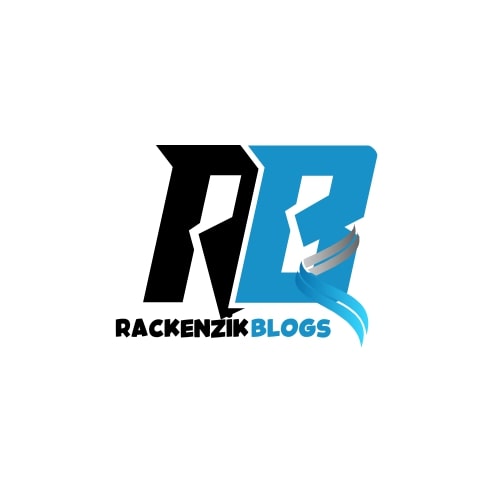