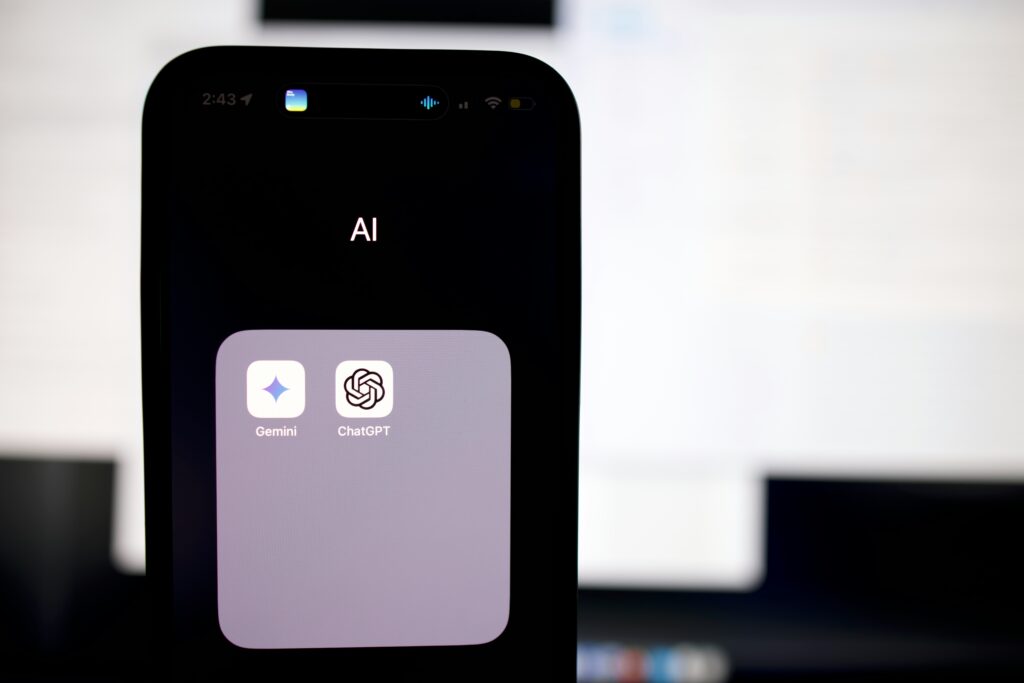
Introduction
Acute ischemic stroke (AIS) remains a leading cause of disability and death. Early, accurate diagnosis is crucial for improving patient outcomes. This blog explores the diagnostic efficacy and capabilities of two powerful AI models, ChatGPT-4o and Claude 3.5 Sonnet, in detecting AIS and assessing their effectiveness in stroke localization and identification.
Why Stroke Diagnosis Needs AI
Timely identification of AIS can make the difference between recovery and lasting damage. Modern medical imaging, especially Diffusion-Weighted Imaging (DWI), plays a central role in diagnosis. As medical data grows more complex, AI steps in to assist radiologists with fast, consistent, and informed interpretations.
This study evaluates how well ChatGPT-4o and Claude 3.5 Sonnet identify AIS using DWI and ADC images.
Diagnostic Efficacy: How the Study Was Conducted
Diagnostic Efficacy: Patient Selection
Researchers analyzed DWI scans from 1,256 patients in a retrospective study. They selected cases with strict inclusion criteria: patients over 18 who showed clinical signs of AIS—such as hemiparesis, aphasia, or facial asymmetry—and confirmed diffusion restriction on DW-MRI.
The study included:
- 55 AIS cases with confirmed diffusion restriction
- 55 healthy controls with no signs of restriction, imaged for unrelated reasons
Radiologists excluded cases with artifacts, patients under 18, or those with intracranial masses.
Diagnostic Efficacy: Image Preparation
Researchers used a 1.5T MRI scanner to acquire DWI and ADC images. They selected the most informative slices, anonymized the DICOM files, and converted them to JPEG format without losing critical detail. They cropped irrelevant areas and randomized the image order before presenting them to the AI models.
Diagnostic Efficacy: Standardized Prompt Design
Each AI model received a structured, three-part prompt:
- “Do these DWI and ADC images show an acute ischemic stroke? Answer Yes or No.”
- “If yes, where is the stroke located? (Right/Left cerebral or cerebellar hemisphere)”
- “If yes, which specific brain lobe or region is affected?”
This consistent approach allowed a fair and focused comparison between the models.
Evaluation Metrics
Researchers measured sensitivity, specificity, diagnostic accuracy, positive/negative predictive value (PPV/NPV), F1 score, and Cohen’s Kappa for agreement with radiologists.
Diagnostic Efficacy: Performance Showdown
Diagnostic Efficacy: Demographics
Both groups (AIS and controls) were statistically similar in age and gender distribution.
Metric | AIS Group (n=55) | Control Group (n=55) | p-Value |
---|---|---|---|
Female | 25 (45.5%) | 25 (45.5%) | 1.0 |
Male | 30 (54.5%) | 30 (54.5%) | |
Age (mean ± SD) | 73.11 ± 11.43 | 72.75 ± 10.75 | 0.90 |
Diagnostic Accuracy
- ChatGPT-4o identified all AIS cases correctly (100% sensitivity) but misclassified nearly all healthy cases as strokes (3.6% specificity).
- Claude 3.5 Sonnet achieved 94.5% sensitivity and 74.5% specificity, resulting in 84.5% overall accuracy.
Metric | ChatGPT-4o (%) | Claude 3.5 Sonnet (%) |
---|---|---|
Accuracy | 51.8 | 84.5 |
Sensitivity | 100 | 94.5 |
Specificity | 3.6 | 74.5 |
PPV | 50.9 | 78.8 |
NPV | 100 | 93.2 |
F1 Score | 67.5 | 85.9 |
Answer Accuracy
Claude 3.5 Sonnet outperformed ChatGPT-4o in correctly answering all three diagnostic questions.
Model | Fully Correct Responses (%) | Partially/Incorrect Responses (%) |
---|---|---|
ChatGPT-4o | 7.3 | 92.7 |
Claude 3.5 Sonnet | 30.9 | 69.1 |
Agreement with Radiologists
Claude 3.5 Sonnet showed strong alignment with expert opinion.
Model | Cohen’s Kappa (κ) |
---|---|
ChatGPT-4o | 0.036 (Slight) |
Claude 3.5 Sonnet | 0.691 (Substantial) |
Hemispheric and Specific Localization
Claude 3.5 Sonnet not only identified the hemisphere more accurately but also pinpointed the exact brain region with better precision.
Model | Correct Hemisphere (%) | Specific Region (%) |
---|---|---|
ChatGPT-4o | 32.7 | 7.3 |
Claude 3.5 Sonnet | 67.3 | 30.9 |
Challenges and Limitations
Despite impressive performance, both models struggle with false positives and false negatives, limiting their standalone clinical use. Their stochastic nature can lead to inconsistent answers.
Ethical concerns also persist—transparency, data privacy, and clinical accountability must be addressed before large-scale adoption.
The Road Ahead: AI in Stroke Diagnosis
The results suggest a promising future for Large Vision-Language Models (LVLMs) in stroke detection. Continued training on medical imaging and refined prompt engineering could significantly boost their reliability.
With further development, AI can reduce diagnostic delays, support radiologists, and enhance stroke care—especially in regions with limited access to expert imaging review.
Conclusion
Claude 3.5 Sonnet clearly outperformed ChatGPT-4o in this study. It demonstrated better accuracy, agreement with radiologists, and localization capabilities. While both models offer promise, Claude 3.5 Sonnet currently provides the most dependable results for AIS detection.
To maximize AI’s clinical value, developers must continue refining model precision and minimizing diagnostic errors. With the right safeguards and improvements, AI will become an essential ally in modern stroke diagnostics.
References
Koyun, M., & Taskent, I. (2025). Evaluation of Advanced Artificial Intelligence Algorithms’ Diagnostic Efficacy in Acute Ischemic Stroke: A Comparative Analysis of ChatGPT-4o and Claude 3.5 Sonnet Models. Journal of Clinical Medicine, 14(2), 571. https://doi.org/10.3390/jcm14020571
License
This blog post is licensed under a Creative Commons Attribution 4.0 International License (CC BY 4.0). You are free to share, copy, redistribute, and adapt the content, provided appropriate credit is given.