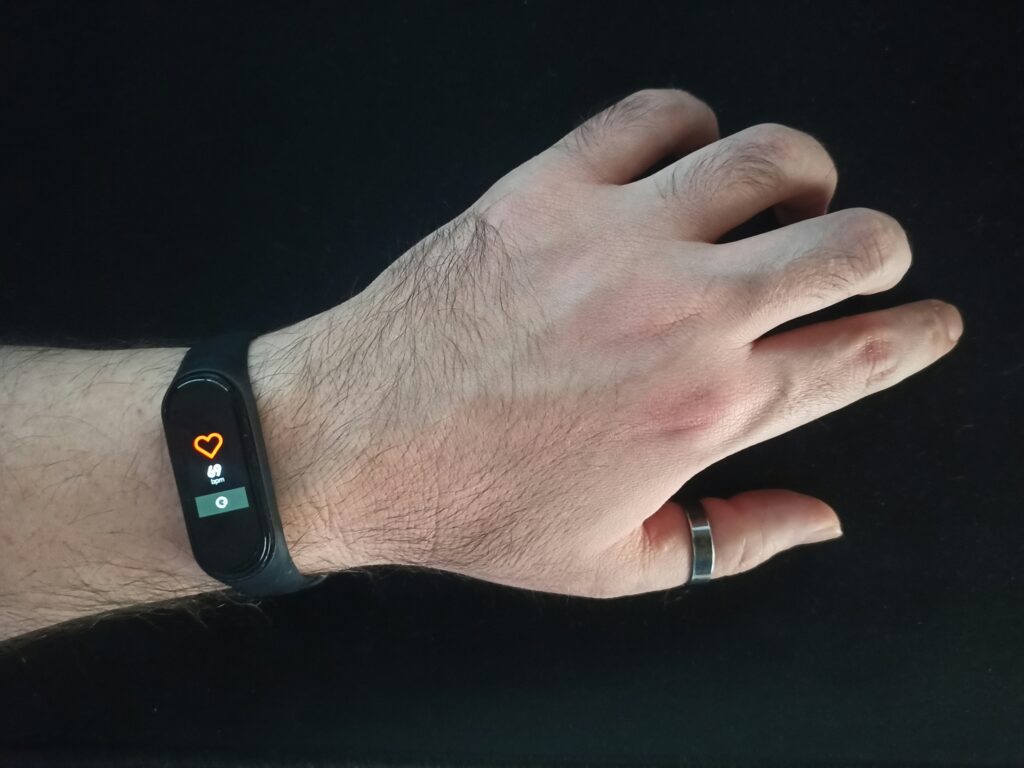
Introduction
Human movement, postural balance, and autonomic responses intricately interact to regulate daily activities efficiently. Variations in heart rate variability (HRV), an established biomarker of autonomic nervous system (ANS) regulation, reflect a physiological interplay between stress responses and motor control adaptations. Chronic stress disrupts ANS functionality, leading to altered HRV patterns that influence postural stability and increase fall risk vulnerability.
Understanding the correlation between HRV fluctuations and autonomic dysfunction enables AI-driven predictive analysis, enhancing early detection mechanisms for postural instability and physiological stress markers. Machine learning models such as unsupervised clustering and supervised classification systematically categorize physiological stress responses, facilitating real-time monitoring of HRV-based autonomic dysregulation.
This blog investigates the impact of daily activities on HRV-modulated stress detection and balance control, examining AI-powered physiological assessments and predictive analytics to optimize fall risk prevention strategies.
Understanding Physiological Responses During Daily Activities
HRV as an Indicator of Autonomic Regulation in Daily Activities
HRV represents a quantifiable measure of autonomic nervous system adaptability, influencing stress regulation and motor function. The sympathetic-parasympathetic balance, often disrupted by chronic stress, impacts postural equilibrium and physiological homeostasis.
HRV Parameter | Physiological Significance | Implication on Stress and Balance |
---|---|---|
RR Intervals | Time between consecutive heartbeats | Decreased intervals indicate elevated sympathetic activation |
SDNN | Standard deviation of normal RR intervals | Low SDNN associated with autonomic dysfunction |
RMSSD | Short-term HRV variability | Decline signifies impaired vagal tone |
LF/HF Ratio | Balance between sympathetic and parasympathetic activity | Increased ratio suggests stress-induced imbalance |
The mechanistic relationship between ANS regulation and postural balance is mediated through central and peripheral autonomic processing. Dysregulated sympathetic activation alters vestibular function, disrupting motor coordination and stability, exacerbating fall risk potential.
AI and Machine Learning in Stress and Fall Risk Detection During Daily Activities
Machine Learning Models for HRV Analysis in Daily Activities
AI-driven autonomic analysis applies machine learning to extract hidden physiological patterns indicative of stress responses and postural instability.
Machine Learning Technique | HRV-Based Application | Outcome |
---|---|---|
K-Means Clustering | Unsupervised learning to categorize physiological states | Distinct stress level classification |
Gradient Boosting Classifier | Fall risk prediction based on HRV signals | Achieves 95.45% accuracy in balance loss detection |
Neural Networks | Processing HRV fluctuations for deeper physiological insights | Increased adaptability for personalized monitoring |
HRV-based AI modeling enables predictive assessments, refining classification accuracy for stress and postural regulation monitoring.
Methodology: AI-Driven Data Analysis on Daily Activities
Data Collection and Processing in Daily Activities
Fourteen participants wore Polar H10 heart rate monitors, collecting inter-beat interval (IBI) signals during Berg Balance Scale (BBS) activities. The data preprocessing pipeline included median filtering for motion artifact removal, Butterworth low-pass filtering for noise reduction, and feature extraction for HRV spectral and time-domain parameters.
HRV patterns were categorized using unsupervised clustering, and postural balance was classified using supervised learning algorithms.
Working Mechanisms of AI-Based Stress and Balance Monitoring
AI-Driven Autonomic Dysregulation Detection
AI models analyzed HRV-derived physiological states across daily movement scenarios, detecting stress-related autonomic imbalance.
HRV Feature | Significance in Stress Analysis | Role in Fall Risk Detection |
---|---|---|
LF/HF Ratio | Evaluates sympathetic dominance | Elevated ratio correlates with instability |
RMSSD | Quantifies parasympathetic fluctuations | Low RMSSD signals autonomic dysfunction |
SDNN | Measures overall HRV variability | Reduced SDNN linked to impaired postural control |
Machine learning enhances HRV-based clinical applications, developing predictive biomarkers for stress and motor deficiencies.
Results and Findings
AI’s Contribution to Fall Risk Detection
Gradient Boosting achieved 95.45% classification accuracy, surpassing traditional models in fall risk prediction reliability.
Metric | Gradient Boosting Accuracy |
---|---|
Precision | 93.10% |
Recall | 85.71% |
F1 Score | 85.71% |
Overall Accuracy | 95.45% |
AI-driven stress monitoring refines real-time autonomic assessments, supporting wearable integration for fall prevention technologies.
Discussion: Theoretical Implications and AI’s Role in Preventive Healthcare
AI and Autonomic Neurovisceral Integration
HRV-based AI modeling confirms neurovisceral integration theory, establishing links between ANS regulation and physiological stress adaptation, HRV reductions and increased fall risks, and AI’s potential in detecting autonomic dysfunction in early stages.
Gradient Boosting and K-Means clustering optimize stress detection frameworks, strengthening predictive postural stability analytics.
Conclusion
Conclusion
AI’s Role in Advancing Stress and Fall Risk Monitoring
The integration of artificial intelligence in physiological monitoring represents a transformative shift in daily activity analysis and healthcare. By leveraging machine learning techniques, researchers have successfully extracted meaningful insights from heart rate variability (HRV) patterns, enabling real-time predictions of stress levels and fall risks. The findings of this study highlight the capability of AI-driven models to detect autonomic imbalances, reinforcing their potential role in early intervention strategies for individuals vulnerable to stress-induced balance impairments.
Theoretical Significance of AI-Based Fall Risk Prediction
From a theoretical standpoint, this study reaffirms the neurovisceral integration model, which posits that autonomic dysregulation contributes to both psychological stress responses and motor instability. The correlation observed between HRV reductions and postural disturbances aligns with existing literature on sympathetic dominance and altered motor coordination. AI’s ability to process these physiological variations in real-time offers clinical applications in wearable technologies, ensuring continuous autonomic monitoring and proactive intervention for balance control.
Methodological Contributions and Model Performance Evaluation
The methodological framework employed in this study combines unsupervised clustering for physiological state categorization and supervised classification for fall risk prediction, demonstrating a multi-dimensional approach to autonomic assessment. The superior performance of Gradient Boosting classifiers, achieving 95.45% accuracy, underscores the efficacy of ensemble learning techniques in handling HRV-based autonomic variability. The integration of machine learning in stress detection and balance monitoring paves the way for more accurate, scalable, and adaptive AI models in health monitoring applications.
Implications for Future Research and Technological Advancements
Given the promising results of AI-driven HRV-based physiological monitoring, future studies should aim to expand datasets, incorporating diverse demographic groups with varying autonomic profiles. Large-scale validation of AI models is essential to ensure generalizability and robustness across populations. Advancements in biofeedback mechanisms, digital twin simulations, and AI-enhanced wearable systems can further refine real-time stress management and fall prevention strategies, ultimately contributing to autonomous health regulation.
Final Thoughts
This study emphasizes the critical intersection between AI, physiology, and healthcare, demonstrating how machine learning innovations are reshaping stress detection and postural balance monitoring. As AI technologies evolve, integrating real-time physiological analytics into wearable health solutions will enable proactive stress regulation and fall prevention efforts, offering new possibilities for enhanced quality of life, personalized health monitoring, and clinical decision-making.
Reference: Messaoud, I.B., & Thamsuwan, O. (2025). Heart Rate Variability-Based Stress Detection and Fall Risk Monitoring During Daily Activities: A Machine Learning Approach. Computers, 14(2), 45. https://doi.org/10.3390/computers14020045.
Creative Commons License: This article is distributed under the Creative Commons Attribution (CC BY) license, which allows unrestricted use, distribution, and reproduction in any medium, provided that the original authors are credited. More details can be found at: https://creativecommons.org/licenses/by/4.0/.