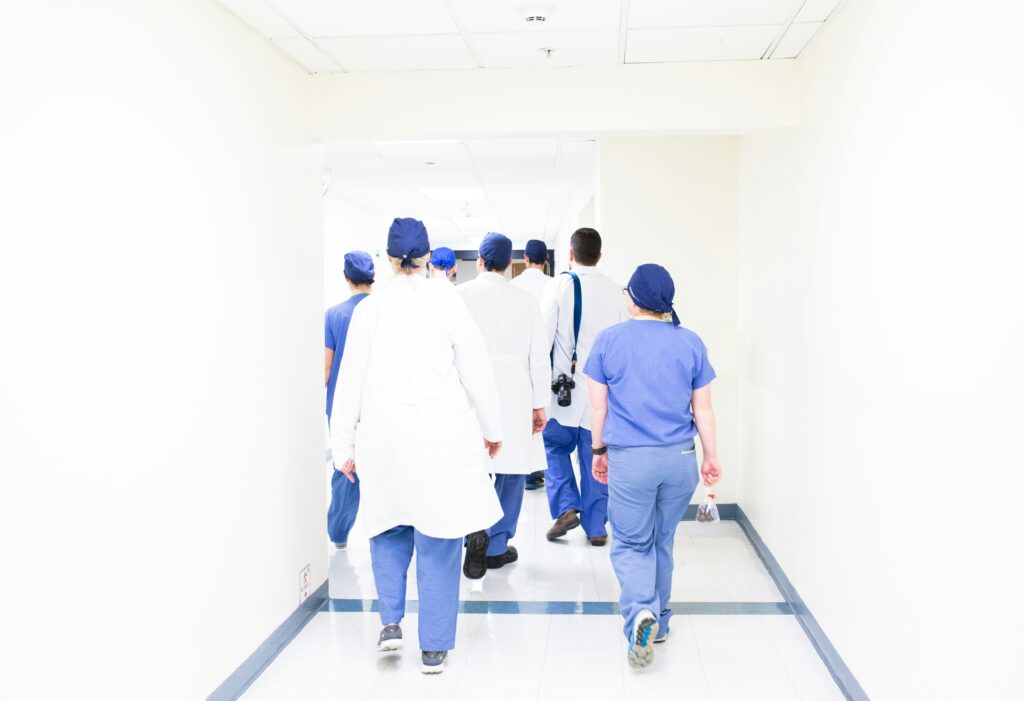
1. Introduction
Defining Precision Medicine and Its Growing Role
Precision medicine is revolutionizing healthcare by tailoring diagnostics and treatments to individual genetic, environmental, and lifestyle factors. Unlike traditional therapies that apply a one-size-fits-all approach, precision medicine customizes treatments for better patient outcomes.
The Shift from Generalized Treatments to Personalization
Historically, medical decision-making relied on standardized treatment protocols. However, advances in high-throughput DNA sequencing and biomarker identification now enable individualized therapies based on molecular insights. Companies like CD Genomics provide cutting-edge genomic sequencing solutions, supporting precision medicine by identifying genetic variants that influence drug response and disease susceptibility. Their sequencing technologies help researchers and clinicians optimize treatment strategies, making personalized medicine more accessible and effective.
In Silico Techniques Enhancing Precision Medicine
Computational modeling, AI, and bioinformatics play a key role in accelerating drug discovery, clinical pharmacology, and patient-specific treatment strategies. These techniques reduce development time and costs, improving healthcare efficiency.
2. Understanding In Silico Approaches in Precision Medicine
Computational Models and Simulations in Precision Medicine for Drug Discovery
In silico methods incorporate biological modeling, high-throughput screening, and AI-driven analytics to optimize drug formulations and dosing regimens. This enables researchers to test therapeutic hypotheses before moving to human trials.
The Role of AI, Machine Learning, and Bioinformatics
Machine learning models analyze big data from genomics, proteomics, and electronic health records (EHRs) to predict patient-specific drug responses and disease progressions. AI-driven simulations enhance biomarker identification for precision medicine applications.
Integration with Genomics, Proteomics, and Pharmacometrics
Modern computational tools help integrate omics data to understand drug interactions at a molecular level. Genomic sequencing, protein analysis, and pharmacokinetic modeling collectively improve personalized treatment strategies.
Methodology: In Silico Techniques in Precision Medicine Drug Development
1. High-Throughput DNA Sequencing Technologies & Clinical Decision-Making
Advancements in high-throughput DNA sequencing technologies have drastically changed the landscape of drug development and precision medicine. These sequencing techniques allow scientists to rapidly analyze and decode the human genome, providing critical insights into genetic variations that influence disease progression, drug metabolism, and individual responses to medications.
In precision medicine, genomic data serves as a foundation for clinical decision-making. By integrating sequencing technologies with bioinformatics platforms, researchers can identify:
- Genetic mutations related to drug efficacy and toxicity.
- Pharmacogenomic markers that help predict drug response variability across patient populations.
- Disease-associated genetic risk factors, aiding in early disease detection and prevention strategies.
Additionally, in silico approaches complement sequencing efforts by modeling genetic interactions to predict potential drug targets, reducing the reliance on traditional trial-and-error drug development approaches.
2. Utilizing Pharmacometrics in Precision Medicine for Optimized Drug Dosages
Pharmacometrics is an essential computational tool in drug development that quantifies drug interactions, helping researchers predict optimal dosage and treatment plans tailored to individual patient needs.
Through mathematical modeling, pharmacometrics assesses:
- Pharmacokinetics (PK) – How the body absorbs, distributes, metabolizes, and eliminates a drug.
- Pharmacodynamics (PD) – The drug’s effects on biological systems and its therapeutic impact.
Using physiologically-based pharmacokinetic (PBPK) models, researchers simulate drug behavior in different patient populations, allowing for:
- Dose individualization for different demographics (e.g., pediatric, elderly, and patients with renal impairment).
- Predicting drug-drug interactions (DDIs) to reduce adverse effects in patients taking multiple medications.
- Optimizing therapy durations, ensuring maximum drug efficacy while minimizing toxicity risks.
📌 Table: Key Applications of Pharmacometrics in Precision Medicine
Application | Description |
---|---|
Predicting drug efficacy | Models drug absorption and therapeutic response. |
Identifying optimal dosage | Provides individualized treatment plans based on PK/PD. |
Minimizing toxicity risks | Reduces risk of drug-related side effects by optimizing dosing. |
Improving trial design | Helps refine clinical trial protocols with data-driven predictions. |
Drug-drug interaction analysis | Predicts potential DDIs for safer polypharmacy treatments. |
Through advanced machine learning (ML) integration, pharmacometric models can continuously analyze real-world patient data to refine treatment recommendations based on evolving patient responses.
3. Digital Health Tools: Wearable Technology, Real-Time Monitoring & EHRs
With the digital revolution, precision medicine increasingly relies on real-time data collection via digital health technologies, including wearable devices and electronic health records (EHRs).
Wearable Technology
Wearables such as smartwatches, continuous glucose monitors, and biosensors provide continuous physiological monitoring, enabling:
- Real-time tracking of vital signs (heart rate, oxygen levels, blood pressure).
- Patient-specific medication adherence monitoring.
- Early detection of adverse drug reactions via biometric data collection.
Electronic Health Records (EHRs)
EHR systems store large-scale patient data, integrating:
- Genomic profiles with historical patient records.
- Pharmacogenomic testing results to refine therapy choices.
- AI-driven clinical decision support systems (CDSS) to optimize precision dosing and improve physician decision-making.
📌 Table: Digital Health Innovations Enhancing Drug Development
Technology | Function in Precision Medicine |
---|---|
Wearable devices | Monitors real-time patient data for continuous treatment optimization. |
AI-driven EHRs | Integrates patient history with pharmacogenomic insights. |
Smart sensors | Provides biometric data on drug reactions and patient health metrics. |
Remote patient monitoring | Tracks therapy response outside clinical settings. |
By integrating in silico modeling, pharmacometric predictions, and digital health technologies, precision medicine achieves a dynamic, patient-centric approach in drug development.
4. Working of In Silico Models in Precision Medicine
Application of Physiologically-Based Pharmacokinetic (PBPK) Models
PBPK models provide a mechanistic framework to predict drug absorption, distribution, metabolism, and excretion (ADME) in different patient populations. These models integrate biological, anatomical, and physiological variables to ensure individualized drug regimens, essential for precision medicine.
PBPK models enable:
- Personalized drug dosing for pediatric, elderly, and renal-impaired patients.
- Simulation of drug-drug interactions (DDIs) to mitigate adverse effects.
- Prediction of drug exposure in pregnancy and disease states for tailored treatments.
The attached research paper highlights the importance of PBPK simulations in reducing clinical trial costs and optimizing drug formulations, ensuring effective therapy with fewer side effects.
Example: PBPK in Oncology Treatments PBPK models have significantly impacted oncology, improving the dosing of chemotherapeutic agents based on individual patient biomarkers. The integration of tumor-specific PBPK models enhances prediction accuracy for drug response.
Machine Learning Algorithms for Predictive Healthcare
Machine learning (ML) is revolutionizing precision medicine, processing large datasets, and identifying patterns in drug development. ML-driven in silico models enhance:
- Predictive pharmacology, optimizing drug efficacy using past clinical data.
- Automated biomarker identification, allowing precise disease profiling.
- Real-time patient monitoring, refining therapy adjustments dynamically.
The attached research paper emphasizes AI-based predictive models, improving the efficiency of drug development pipelines by using deep learning frameworks. ML models uncover relationships between genomic variations and drug responses, leading to personalized treatments.
Example: ML for Drug Repurposing ML algorithms are used to screen existing drugs against novel diseases, identifying potential therapies with optimized treatment strategies.
Integration of Omics Data for Patient-Specific Drug Response
Omics technologies such as genomics, proteomics, metabolomics, and transcriptomics play a central role in precision medicine. These fields provide deep molecular insights into disease mechanisms, allowing tailored treatments.
Key applications of omics in drug development:
- Pharmacogenomics enables genetic biomarker-driven therapy selection.
- Proteomics analysis refines targeted therapeutic approaches in cancer treatment.
- Metabolomics insights predict drug toxicity and metabolism variations.
Table: Contribution of Omics in Precision Medicine
Omics Field | Application in Drug Development |
---|---|
Genomics | Identifies genetic variations influencing drug response |
Proteomics | Maps protein interactions to optimize therapy |
Metabolomics | Detects metabolic fluctuations affecting drug efficacy |
Transcriptomics | Analyzes gene expression patterns for precision therapy |
The research paper discusses multi-omics integration, highlighting its ability to refine patient-specific treatment pathways.
5. Results: Breakthroughs and Real-World Applications
Success Stories in Oncology and Rare Disease Treatment
Precision medicine has transformed cancer care, ensuring targeted therapies based on molecular markers. Genomic sequencing has facilitated customized cancer treatment, leading to higher survival rates.
📌 Example: Precision Medicine in Oncology Cancer treatments such as immunotherapy and biomarker-driven chemotherapy have improved patient outcomes, as documented in the research paper.
Rare diseases also benefit from in silico models, identifying genetic mutations linked to disorders such as cystic fibrosis and muscular dystrophy.
FDA-Approved Drugs Utilizing Precision Medicine Approaches
Precision medicine has contributed to the development of FDA-approved drugs targeting genetic-specific diseases.
Table: FDA-Approved Precision Medicine Treatments
Therapeutic Indication | FDA-Approved Drugs |
---|---|
Cancer | Abecma (Multiple Myeloma), Lumakras (Lung Cancer) |
Rare Diseases | Amondys (Muscular Dystrophy), Nexviazyme (Pompe Disease) |
Other Diseases | Cabenuva (HIV-1), Leqvio (Hypercholesterolemia) |
According to the research paper, biomarker-driven approvals enhance individualized therapy success.
Challenges in Large-Scale Implementation
Despite its potential, precision medicine faces several barriers to widespread adoption:
1. Ethical Concerns
- Patient data privacy in genomic medicine.
- Risk of genetic discrimination in insurance policies.
2. Data Privacy Issues
- Storage of genetic information in cloud-based systems raises security concerns.
- Regulatory challenges in cross-border genomic data sharing.
3. Cost and Accessibility
- High cost of omics technologies hinders global adoption.
- Need for healthcare systems to invest in precision medicine infrastructure.
The attached paper explores these challenges, emphasizing policy and regulation changes needed for successful implementation.
6. Future Prospects of Precision Medicine and In Silico Technologies
Evolution of Adaptive Clinical Trials and Biomarker-Driven Treatments
Adaptive clinical trials have emerged as innovative approaches, enabling dynamic modifications to trial protocols based on interim findings. These trials allow:
- Early termination of ineffective treatments to minimize patient exposure to suboptimal therapies.
- Real-time patient stratification, optimizing biomarker-driven precision treatments.
- Integration of multi-omics data into drug trials to enhance therapeutic predictability.
As described in the research paper, biomarker identification through computational models enhances patient screening for targeted therapy selection, ensuring efficient drug development.
Example: Biomarker-Driven Oncology Trials Cancer treatments are increasingly tailored using genetic biomarkers, allowing targeted therapies for subpopulations based on tumor-specific mutations.
Expanding AI-Driven Solutions for Predictive Healthcare
Artificial intelligence (AI) continues to redefine medical research, particularly in data-driven diagnostics and drug discovery. AI-driven applications in precision medicine include:
- Predictive analytics for disease prevention using real-world patient data.
- Deep learning models for early cancer detection through radiomic imaging biomarkers.
- Automated drug repurposing strategies to discover novel therapeutic indications.
The research paper highlights machine learning (ML) algorithms, which analyze large-scale genomic datasets, improving the efficiency of precision medicine applications.
Table: AI in Precision Medicine Advancements
AI Application | Impact in Precision Medicine |
---|---|
Genomics-based AI screening | Accelerates disease biomarker identification |
AI-driven drug repurposing | Speeds up drug discovery for rare diseases |
Clinical decision support systems (CDSS) | Optimizes precision dosing for patient subgroups |
Predictive healthcare analytics | Enables early disease detection and intervention |
With continued improvements in computational power, AI-driven healthcare solutions are set to reshape medical diagnostics and therapy personalization.
Overcoming Regulatory and Infrastructural Barriers for Broader Adoption
Despite its promise, precision medicine encounters regulatory hurdles and infrastructural challenges that must be addressed for mainstream implementation.
Key challenges include:
- Standardization of genomic testing protocols across healthcare systems.
- Ethical concerns surrounding genetic data privacy and security.
- Integration of AI-driven clinical decision tools into healthcare frameworks.
The research paper emphasizes the role of global collaborations between regulatory agencies, pharmaceutical industries, and AI researchers in overcoming these barriers.
Example: International Regulatory Initiatives Organizations such as the FDA and EMA are working towards harmonized frameworks for genomic data use in precision medicine trials.
Conclusion
Recap of Precision Medicine’s Impact on Drug Development and Treatment Strategies
Precision medicine empowered by AI and in silico techniques has transformed:
- Drug discovery and clinical trial efficiency.
- Patient-centric treatment personalization.
- Targeted therapies for complex diseases.
The Promising Future of In Silico Methodologies in Advancing Personalized Medicine
AI-driven pharmacometric models, PBPK simulations, and omics data integration will continue to enhance precision medicine applications.
Call-to-Action: Encouraging Further Research and Awareness in Precision Healthcare
As computational and genomic technologies evolve, fostering multidisciplinary collaborations will ensure accessibility and ethical implementation of precision medicine innovations
Reference: Marques, L., Costa, B., Pereira, M., Silva, A., Santos, J., Saldanha, L., Silva, I., Magalhães, P., Schmidt, S., & Vale, N. (2024). Advancing Precision Medicine: A Review of Innovative In Silico Approaches for Drug Development, Clinical Pharmacology and Personalized Healthcare. Pharmaceutics, 16(332). https://doi.org/10.3390/pharmaceutics16030332
License: This article is published under the Creative Commons Attribution (CC BY) license: CC BY 4.0