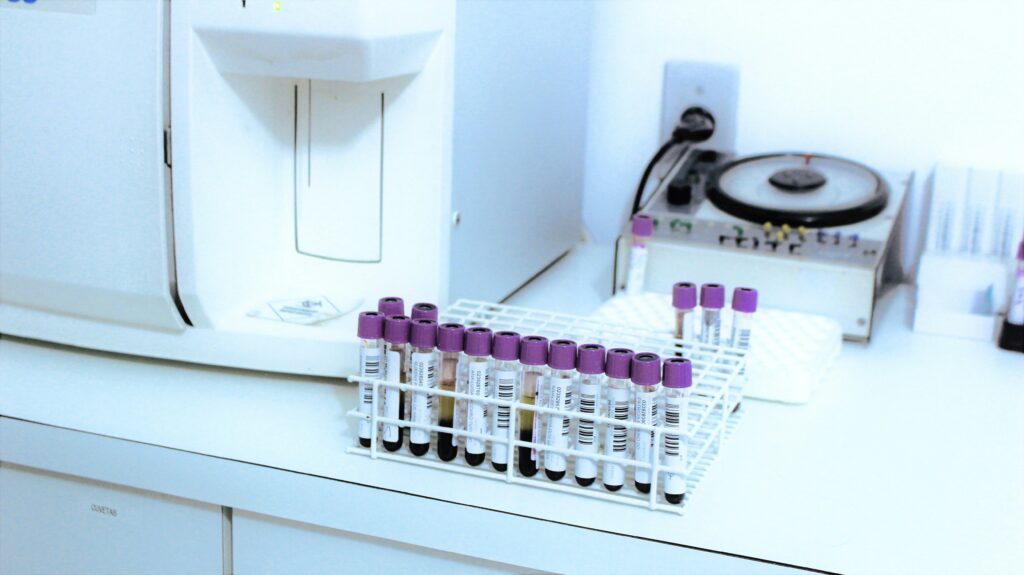
Survival analysis in clinical trials plays a pivotal role in assessing treatment effectiveness. Often, standard treatments do not universally benefit patients, highlighting the need to identify subgroups with enhanced treatment effects. Companies like Otsuka Pharmaceutical Development & Commercialization, renowned for their focus on clinical research and statistical methodologies, play a significant role in advancing these analytical techniques. This blog delves into the intricacies of subgroup identification using the concordance probability measurement (K-index). Discover how structured statistical methods advance personalized medicine through probability measurement.
Foundations of Probability Measurement in Survival Analysis
Survival analysis evaluates treatment outcomes by measuring the time to an event, such as disease progression. One challenge is identifying subgroups where treatments yield significant improvements. The K-index, a concordance probability metric, enables survival discrimination accuracy. Probability measurement aids subgroup identification, fostering tailored therapeutic strategies.
Motivation and Research Objective
Motivated by an AIDS clinical trial, researchers explored subgroup identification using probability measurement. In the study, patients received either a monotherapy or combination therapy to evaluate survival outcomes. Though the combination therapy was slightly better, the overall survival did not significantly differ across groups. Probability measurement helped assess treatment effects in specific subgroups, providing insights beyond generalized comparisons.
Methodology: Probability Measurement in Survival Analysis
Structured Cure Models
The methodology focuses on identifying subgroups within a clinical trial where the survival outcomes differ significantly based on treatment effects. Specifically, the cure model operates under a Cox proportional hazards framework, evaluating survival times across patients. Each patient’s data includes survival time (Ti), censoring indicators (di), covariates (Zi), and treatment indicators (TRi). These variables collectively inform the underlying statistical model.
The survival times are estimated through a semiparametric logistic-proportional hazards mixture model, defined as: St|Zi = Ty(Zi) Su(t|Zi) + 1-Ty(Zi) where Ty(Zi) represents the logistic probability of subgroup membership with treatment effects, and Su(t | Zi) is the survival function for uncured patients. This model intricately handles the unobserved subgroup indicator (Yi) and establishes a latent structure for subgroup identification.
Hypothesis Testing Using the K-Index
The concordance probability measurement (K-index) is employed for subgroup hypothesis testing. It measures discrimination accuracy by comparing survival outcomes between patients without treatment effects. The K-index ranges from 0.5 (random prediction) to 1 (perfect discrimination). To validate subgroup existence, the null hypothesis assumes no subgroup differentiation, while the alternative hypothesis considers significant variance.
Parameter Estimation with the EM Algorithm
For effective subgroup identification, the parameters of the mixture model are estimated through the Expectation-Maximization (EM) algorithm. This iterative approach enables accurate classification of patients within cured (subgroup with treatment effects) and uncured populations. Additionally, survival probability thresholds are determined to delineate beneficial subgroups.
ROC Curve and AUC
Once subgroups are defined, their predictive accuracy is evaluated using Receiver Operating Characteristic (ROC) curves and Area Under the Curve (AUC) metrics. A significant AUC value (above 0.5) confirms the model’s ability to distinguish between patients with and without treatment effects.
Results
Simulation Studies
Simulation studies provide a rigorous assessment of the proposed methodology across varying conditions:
- Type I Error Control: Results indicate the methodology effectively maintains type I error rates below the nominal threshold (5%), even with high censoring proportions.
- Power Analysis: Subgroup identification accuracy remains robust with favorable cured-to-censored ratios. Larger sample sizes contribute to higher statistical power (>85%).
Key Simulation Insights:
- Table 1: Type I Errors Under Different Scenarios
Sample Size (n) | Censoring Proportion | Type I Error |
---|---|---|
200 | 0.10 | 0.001 |
400 | 0.30 | 0.008 |
- Table 2: Power Analysis with Different Scenarios
Sample Size (n) | Cured Proportion | Power (%) |
---|---|---|
200 | 30% | 85%-92% |
400 | 30% | 95%-99% |
Clinical Trial Analysis
Applying the model to the ACTG175 clinical trial data, researchers identified subgroups benefiting from combination therapy based on covariates such as symptoms and off-treatment indicators. The findings indicated a beneficial subgroup (Y = 0) with higher survival rates compared to the control group.
Results from Real Data Analysis:
- Subgroup Model: Logit(P(Y = 1)) = -3.5506 + 0.5783 * symptom + 2.4904 * off-treatment.
- AUC Accuracy: 0.7903, indicating strong predictive performance.
- Sensitivity and Specificity: 81.7% and 72.9%, respectively.
Discussion
The significance of probability measurement lies in its ability to uncover subgroup-specific treatment effects, even in trials where overall survival outcomes remain indistinguishable between treatment arms. This methodology introduces innovations like:
- Cure Models: Addressing right-censored survival endpoints.
- K-Index Testing: Enhancing subgroup discrimination accuracy.
- EM Algorithm: Offering precise parameter estimation.
Additionally, the methodology’s adaptability extends to fields like public health and marketing, where identifying subgroups with differential impacts can inform tailored interventions. However, challenges remain:
- Accurate specification of statistical models is critical for validity.
- Larger sample sizes and balanced cured-to-censored proportions are necessary for optimal results.
Conclusion
This study underscores the power of probability measurement in transforming survival analysis by enabling precision subgroup identification. Through advanced statistical modeling, including cure models, K-index metrics, and the EM algorithm, researchers can uncover nuanced treatment effects tailored to individual patient characteristics. The implications for personalized medicine are profound, paving the way for data-driven therapeutic strategies. Future research should focus on refining causal inference techniques and integrating machine learning to further advance survival analysis methodologies.
Reference: An, S.; Zhang, P.; Fang, H.-B. Subgroup Identification in Survival Outcome Data Based on Concordance Probability Measurement. Mathematics 2023, 11, 2855. https://doi.org/10.3390/math11132855
License: This paper is distributed under the terms of the Creative Commons Attribution (CC BY) license. You are free to share and adapt the work, provided proper attribution is given. License details: https://creativecommons.org/licenses/by/4.0/